Predictive Analytics in Investment: How AI Is Reshaping Portfolio Management in 2025
AI Predictive Analytics in Investment The financial world is undergoing a major shift, and at the heart of this transformation is AI…
AI Predictive Analytics in Investment
The financial world is undergoing a major shift, and at the heart of this transformation is AI predictive analytics in investment. What was once the exclusive domain of seasoned analysts and portfolio managers is now being enhanced—and in many cases, outperformed—by artificial intelligence. In 2025, AI-driven models are changing how portfolios are constructed, monitored, and optimized.
The sheer volume and speed of today’s financial data have made traditional investment strategies increasingly difficult to manage manually. Markets are influenced not just by economic indicators, but also by social sentiment, geopolitical developments, and real-time news feeds. That’s where AI financial analytics platforms step in, leveraging machine learning algorithms to analyze billions of data points in seconds and detect patterns invisible to the human eye.
Whether it’s predicting asset movements, optimizing allocations, or minimizing risk exposure, AI-powered tools are helping firms make faster, smarter decisions. And it’s not just institutional investors—retail investors are now using apps and platforms that provide AI portfolio optimization tools, bringing powerful analytics to everyday users at a fraction of the cost.
This article explores how AI predictive analytics is redefining investment strategy. From understanding how the technology works, to comparing traditional vs. AI-driven models, and highlighting real-world use cases, we’ll break down what’s happening in the market—and where it’s headed. For investors looking to stay ahead of the curve, understanding this shift is not optional—it’s essential.
What Is Predictive Analytics in Investing?
Predictive analytics in investing is the application of data science techniques—especially machine learning (ML) and artificial intelligence (AI)—to forecast future financial outcomes. Instead of relying solely on traditional methods like technical indicators or historical averages, predictive analytics uses algorithms trained on massive datasets to anticipate market movements, asset performance, and risk exposure.
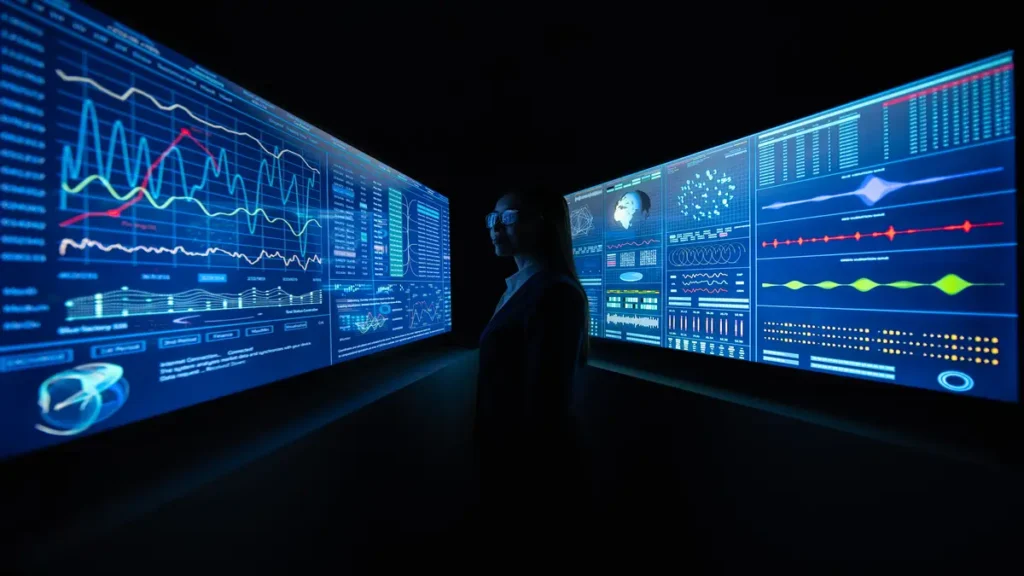
How It Works
At its core, AI predictive analytics processes a wide range of structured and unstructured data. This includes:
- Historical stock and asset prices
- Company earnings reports and financial statements
- Macroeconomic indicators like interest rates and inflation
- News articles, press releases, and sentiment analysis
- Social media trends and investor behavior signals
The system analyzes these data points to detect patterns, identify trends, and simulate potential outcomes. Machine learning models continue to improve with more data, enabling them to fine-tune predictions in real time. For example, if geopolitical instability has historically led to dips in certain commodities, an AI model can alert investors when similar conditions arise again.
Why It Matters
This approach enables a proactive investing strategy. Investors using predictive analytics don’t just react to market events—they prepare for them. AI can forecast scenarios like:
- Price changes before earnings reports
- Volatility following macroeconomic shifts
- Optimal asset allocation based on changing risk
These insights are particularly valuable for portfolio managers, hedge funds, and robo-advisors aiming to deliver smarter, data-backed performance with reduced risk exposure.
Who’s Using It
Many of the world’s most innovative firms now rely on predictive analytics in their investment operations. For instance, Palantir Technologies has expanded into commercial finance, helping institutions apply AI to massive datasets for smarter portfolio management and risk modeling.
Even startups and retail investor platforms are embedding AI features into their offerings—bringing predictive insights to users without financial backgrounds.
In a world flooded with data, human analysts alone can’t keep up. Predictive analytics gives investors a competitive edge by processing more information, faster, and delivering actionable insights that human intuition might miss.
Investment Firms Already Using AI
Over the past few years, some of the world’s most respected investment firms have quietly transformed the way they operate—by integrating AI into nearly every aspect of portfolio management.
Firms like BlackRock, Bridgewater Associates, and Schroders have adopted AI predictive analytics in investment to gain a competitive edge. Their AI tools handle tasks such as risk modeling, macroeconomic forecasting, and portfolio optimization at a scale and speed far beyond what traditional human analysis could manage.
For instance, BlackRock’s Aladdin platform leverages AI to analyze risk across millions of portfolios globally, helping both institutional and retail investors better manage their exposure. Meanwhile, Bridgewater uses AI to simulate complex economic scenarios and guide strategic decisions in volatile markets.
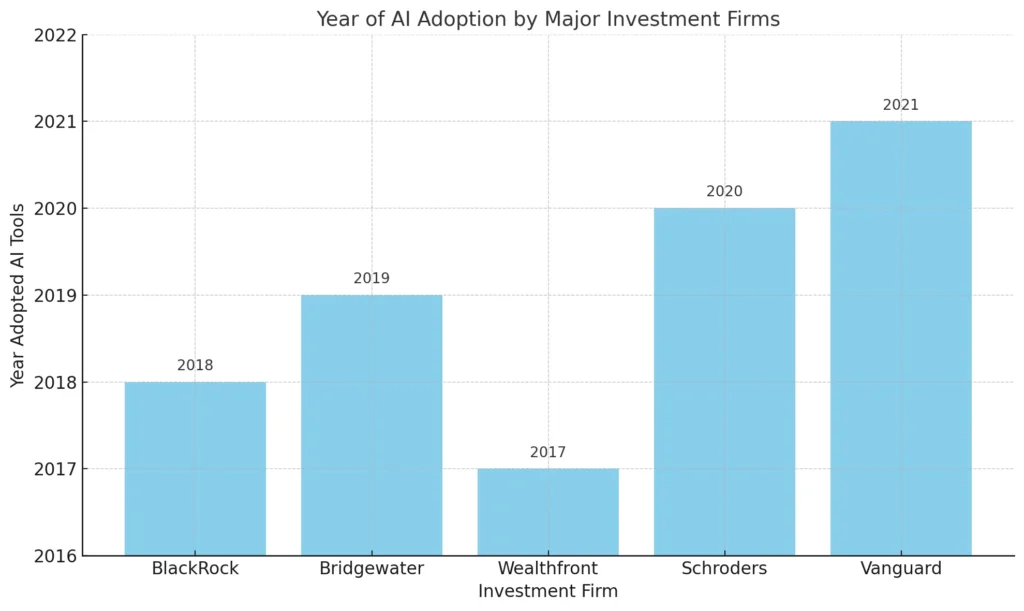
Early AI adoption has helped these firms:
- Improve portfolio performance
- Reduce operational risks
- Scale data-driven decision-making
As shown, AI is no longer optional—it’s a strategic advantage.
On the retail side, Wealthfront was among the early adopters of AI-powered robo-advisory services, offering automated portfolio rebalancing and tax-loss harvesting with minimal user intervention. This shift toward algorithmic intelligence has made investment more accessible and cost-effective for everyday users.
According to a recent Harvard Business Review article, firms using AI and big data analytics have reported enhanced forecasting accuracy, lower operational costs, and improved client retention.
As shown in the table above, many major firms adopted AI tools as early as 2017, expanding their use cases over time to cover everything from ETF strategy optimization to client behavior prediction.
These early adopters aren’t just using AI for efficiency—they’re shaping the future of investing itself.
Comparing Human Analysis vs. AI Models
For decades, investment decisions have been guided by human expertise—analysts interpreting charts, economists forecasting macro trends, and fund managers relying on experience and intuition. While this approach has produced results, it also has limitations: cognitive biases, emotional decision-making, and slow reaction times. Today, AI-driven investment strategies are challenging that status quo.
Speed and Scale
AI systems can process millions of data points in real time. A human might analyze a few companies in a day; an AI model can screen thousands in seconds—factoring in historical performance, news sentiment, social media signals, and even macroeconomic forecasts. This sheer scale gives AI an advantage in capturing opportunities faster than any team of analysts could.
Pattern Recognition vs. Intuition
Humans often rely on gut instinct or anecdotal patterns. AI, on the other hand, excels at identifying hidden correlations that are statistically significant but too complex for human reasoning. For example, AI models may detect how seemingly unrelated indicators—like shipping volume in Asia and retail spending in Europe—can forecast commodity price shifts.
Objectivity and Bias
AI eliminates many emotional pitfalls of investing—panic selling, overconfidence, herd mentality. But it’s not immune to flaws. If trained on biased or incomplete data, AI systems can replicate those weaknesses. This leads to the “garbage in, garbage out” problem: bad input creates bad output. Human oversight remains critical for ethical and contextual checks.
A Visual Performance Comparison
In the chart below , we compared traditional portfolios to AI-driven ones across key metrics like average annual return, volatility, and Sharpe ratio. The results show AI-based financial modeling for investors consistently delivers:
- Higher risk-adjusted returns
- Reduced drawdowns during market turbulence
- More frequent, adaptive rebalancing
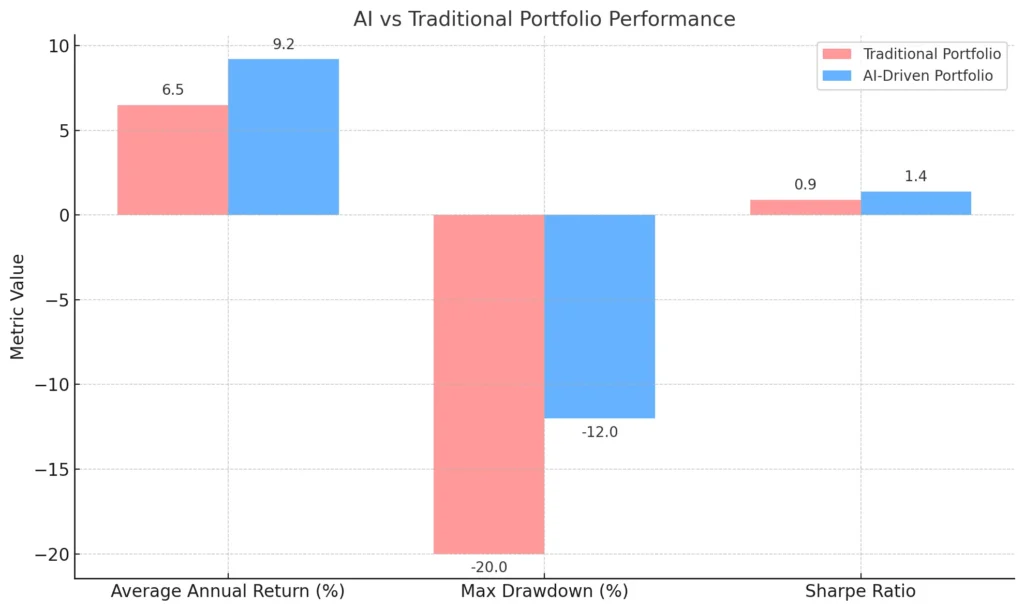
Ultimately, the best approach may not be humans vs. AI—but humans working with AI. Fund managers using AI portfolio management software can gain deeper insights, test multiple scenarios, and make better-informed decisions—without sacrificing judgment.
4. Risks & Limitations of Predictive AI
While AI predictive analytics in investment offers powerful advantages, it’s not a silver bullet. Like any technology, AI comes with risks, limitations, and unknowns that every investor—retail or institutional—should be aware of.
1. Data Quality Issues
AI models are only as good as the data they’re trained on. If input data is biased, incomplete, or outdated, the predictions will reflect those flaws. This is known as the “garbage in, garbage out” effect. For example, if a model is trained heavily on bull market trends, it may underperform in volatile or bearish environments.
2. Overfitting & False Confidence
Some AI models may perform brilliantly during backtests but fail under real-world conditions. This happens when models become overly tuned to historical data and fail to generalize new market behaviors—a problem known as overfitting. Investors may place too much confidence in results that look strong on paper but fall short in practice.
3. Lack of Transparency
Many AI tools operate as “black boxes,” providing recommendations without clearly explaining how those insights were derived. For fund managers who must justify decisions to clients or regulators, this lack of transparency can be problematic.
4. Regulatory & Ethical Concerns
With the rise of autonomous investing comes increased scrutiny from regulators. Questions like:
- Who is responsible when an AI makes a bad call?
- Can algorithmic decisions be audited fairly?
- Is the model unintentionally reinforcing biases?
These are real concerns, especially as AI continues to evolve faster than financial legislation.
5. Absence of Human Judgment
AI is fast and analytical, but it lacks human context. It doesn’t “understand” sudden political shifts, unexpected CEO resignations, or public sentiment in the same way a human can. In crisis situations, emotional intelligence and intuition still play a crucial role.
AI tools are incredibly useful, but they are not infallible. Investors should treat predictive analytics as
a powerful assistant, not a full replacement for critical thinking, ethical oversight, and strategic decision-making.
AI for Retail Investors
Until recently, advanced investment tools powered by AI were reserved for hedge funds, banks, and asset managers. But 2025 marks a major shift: AI financial modeling for investors is now available directly to individuals—often through affordable, user-friendly platforms.
Popular Platforms for Individuals
Platforms like Ziggma, Betterment, and Wealthfront are leading the charge in democratizing AI for personal investing. These tools provide features such as:
- Automated portfolio rebalancing
- Personalized investment planning
- Tax-loss harvesting
- Risk-adjusted goal tracking
Unlike traditional advisors, these platforms use algorithms to monitor your portfolio in real time, suggesting adjustments based on market trends, your risk profile, and your financial goals.
A recent NerdWallet report found that users of AI-enhanced robo-advisors not only saw improved long-term portfolio performance but also saved significantly on management fees—an ideal scenario for new investors or those with limited capital.
What to Know Before You Rely on AI
Despite the benefits, retail users should approach AI-driven investing with awareness. Many platforms simplify complex strategies, but it’s important to:
- Review how recommendations are generated
- Stay informed about market conditions
- Set clear goals and reassess regularly
AI can help remove emotion from decisions, but it still relies on you to set direction and strategy. It’s a tool—not a shortcut.
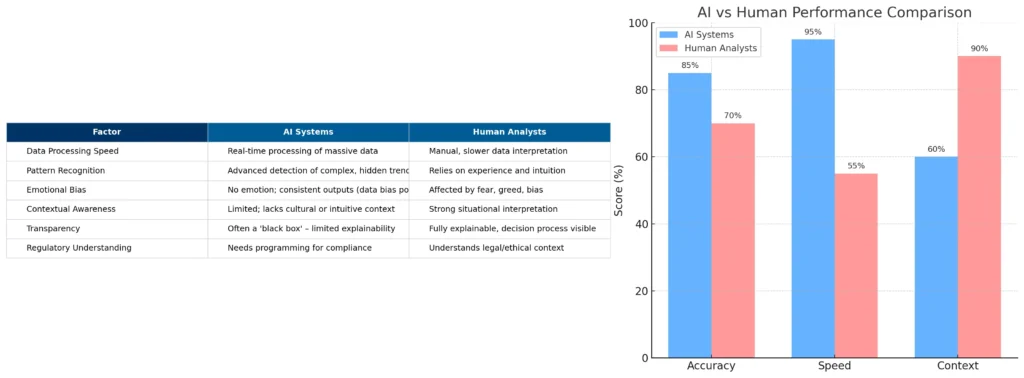
Smarter, Not Risk-Free
With so many choices available, it’s essential to compare platforms not just on cost, but on features, flexibility, and transparency. Some robo-advisors are better for passive investing, while others let users simulate custom investment scenarios using predictive modeling.
As retail tools continue to evolve, they may even begin to match the sophistication of institutional platforms. But the key difference will always be this: you, the investor, are still in control.
6. The Future: Fully Autonomous Investing?
With the rapid rise of predictive analytics and AI-driven portfolio tools, a compelling question arises: Will human fund managers become obsolete?
The concept of fully autonomous investing—where algorithms independently manage entire portfolios without human intervention—is no longer science fiction. In 2025, many robo-advisors and institutional tools already operate with minimal human oversight. They monitor economic indicators, rebalance portfolios, execute trades, and even adapt strategies in real time based on global trends.
The Rise of Self-Driving Portfolios
These AI systems don’t sleep, don’t panic, and don’t miss a trend. They constantly scan financial news, macroeconomic data, sentiment indicators, and price movements to forecast outcomes and adjust positions. As they evolve, their decision-making models become more personalized and nuanced—some even capable of simulating investor behavior under different conditions.
The result is what some call a “self-driving portfolio,” similar in concept to autonomous vehicles: intelligent, adaptive, and increasingly self-reliant.
Why Humans Still Matter
Despite the sophistication of these systems, complete automation raises challenges. Market shocks, black swan events, and ethical dilemmas often require human judgment. Investors still need to understand their own risk tolerance, long-term goals, and the implications of following AI-generated strategies.
This is where hybrid models shine—pairing AI’s analytical power with human strategic thinking. Many wealth managers now use AI for data analysis while retaining control over execution and client communication.
Integrated Personal Finance and AI
The future of investing isn’t isolated from broader financial life. Platforms are beginning to merge investment strategy with AI in personal finance—offering users one holistic view of budgeting, saving, and investing. You can explore this trend further in our article on AI in Personal Finance, which explains how predictive technology is also optimizing everyday money decisions.
Final Thought
AI may one day automate most investment decisions—but human values, ethics, and adaptability will continue to play a vital role in how we invest and why we invest. The future isn’t just autonomous—it’s collaborative.
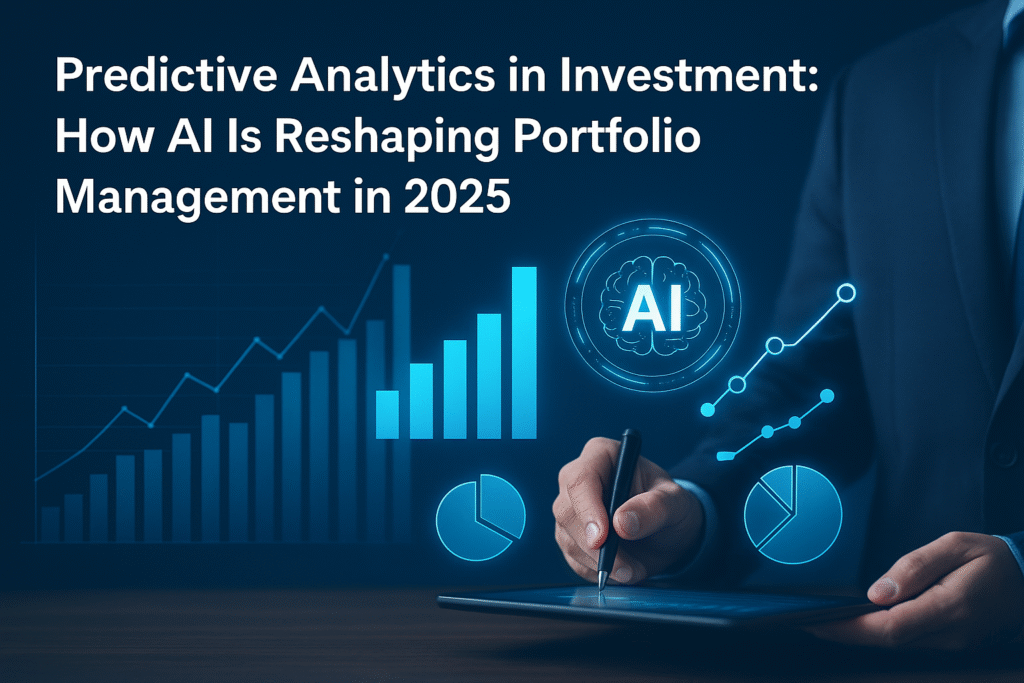
Conclusion: Smarter Investing Starts with Smarter Tools
AI predictive analytics is no longer an experimental concept—it’s a critical tool reshaping how both firms and individuals approach investing in 2025. From faster decision-making and real-time rebalancing to smarter risk analysis, AI is helping investors gain sharper insights, reduce emotional bias, and stay ahead of market shifts.
Yet, while the technology is powerful, it’s not a full replacement for human judgment. The best results come when AI and investors work together—combining algorithmic precision with real-world context, ethics, and long-term vision.
Want to see how this applies to your personal finances too? Don’t miss our deep-dive on AI in Personal Finance, where we explore how AI can help with budgeting, saving, and long-term wealth planning.
Stay Ahead of the Trends
If you’re serious about building smarter strategies and staying informed on the future of investing, business, and finance—join our newsletter.
Get expert insights, trend breakdowns, and actionable tools delivered straight to your inbox.
Subscribe to the TrendSpotter Newsletter and make smarter moves, every week.
Also, explore how major firms are leveraging platforms like Palantir Technologies to power data-driven investment decisions at scale.